The YOLO (You Only Look Once) series is a real-time object detection algorithm that uses convolutional neural network (CNN). It has dramatically shaped the landscape of real-time computer vision. Each iteration of YOLO brings something unique to the table, enhancing the capabilities and applications of object detection. Let’s dive into the evolution of Yolo, details of each major YOLO model, the companies and organizations behind them, and how they contribute to the evolution of AI.
🌐 Timeline of Key YOLO Models and Their Innovators:
1️⃣ Joseph Redmon 🧠:
- YOLO V1 (2016): Redmon introduced the first YOLO model, which revolutionized object detection by framing it as a single regression problem instead of a classification task. This approach allowed YOLO to detect objects in images at unprecedented speeds, making it suitable for real-time applications.
- YOLO V2 & YOLO V3: These versions refined the detection process, improving accuracy with techniques like anchor boxes and multi-scale predictions. YOLO V3 was especially known for its balance between speed and accuracy, making it a benchmark for real-time object detection.
2️⃣ AlexeyAB & WongKinYiu 🔧:
- YOLO V4: Building on Redmon’s work, AlexeyAB introduced YOLO V4, which incorporated advanced techniques like CSPDarknet53 as the backbone, PANet for path aggregation, and various other improvements that significantly boosted detection accuracy while maintaining speed.
- Scaled YOLO V4: WongKinYiu extended YOLO V4 by introducing scaling capabilities, allowing the model to adapt to different sizes depending on the computational resources available.
- YOLO-R & YOLO V7: These versions continued to refine the architecture, focusing on edge-device efficiency without compromising accuracy, and further solidifying YOLO’s role in lightweight, real-time applications.
3️⃣ Ultralytics (Glenn Jocher) 💻:
- YOLO V5: Ultralytics’ YOLO V5 made a significant impact by offering an easier-to-use version of YOLO with extensive support for PyTorch. It’s known for its ease of training, deployment, and integration into various projects. YOLO V5 became the go-to model for many practitioners due to its flexibility and performance.
- YOLO V8: The latest from Ultralytics, YOLO V8, pushes the envelope with state-of-the-art performance, integrating the latest research advancements, and focusing on deployment efficiency in various environments, from cloud to edge devices.
4️⃣ Meituan Technical Team 🌟:
- YOLO V6: Aimed at balancing speed and accuracy, YOLO V6 from Meituan was developed with a focus on real-world applications where inference speed on edge devices is critical. It leverages modern techniques like EfficientNet as the backbone to optimize performance.
- YOLO V6 3.0: This update introduced further refinements in the model architecture, allowing it to perform even better on resource-constrained devices, making it ideal for mobile and embedded applications.
5️⃣ Baidu 🧬:
- PP-YOLO Series: Baidu’s PP-YOLO and its successors (V2 and beyond) are optimized for PaddlePaddle, an AI framework developed by Baidu. PP-YOLO models integrate many of the latest research advancements in object detection, providing a powerful tool for various commercial applications. Baidu’s focus on enhancing speed and accuracy makes PP-YOLO particularly well-suited for industrial AI applications where efficiency is key.
6️⃣ Megvii Technology 🏢:
- YOLOX: Megvii’s YOLOX introduces a new paradigm by decoupling the head of the network into classification and regression branches, improving performance and making it easier to adapt to different tasks. It’s optimized for versatility, robustness, and ease of deployment, particularly in scenarios requiring high accuracy and low latency. YOLOX’s advancements make it a strong contender in the commercial AI space.
7️⃣ Alibaba DAMO Academy 🏛️:
- DAMO YOLO: Alibaba’s DAMO Academy has taken YOLO to new heights with its DAMO YOLO models, which focus on specialized applications requiring high precision. These models leverage PyTorch and Apache licensing and are designed for integration into Alibaba’s vast ecosystem, ensuring scalability and robustness in production environments.
8️⃣ Deci AI 🛠️:
- YOLO-NAS: Deci AI introduces a novel approach with YOLO-NAS, utilizing Neural Architecture Search (NAS) to automatically optimize YOLO models for specific tasks. This results in highly efficient, custom-tailored models that excel in specific applications, providing a significant edge in performance and deployment flexibility.
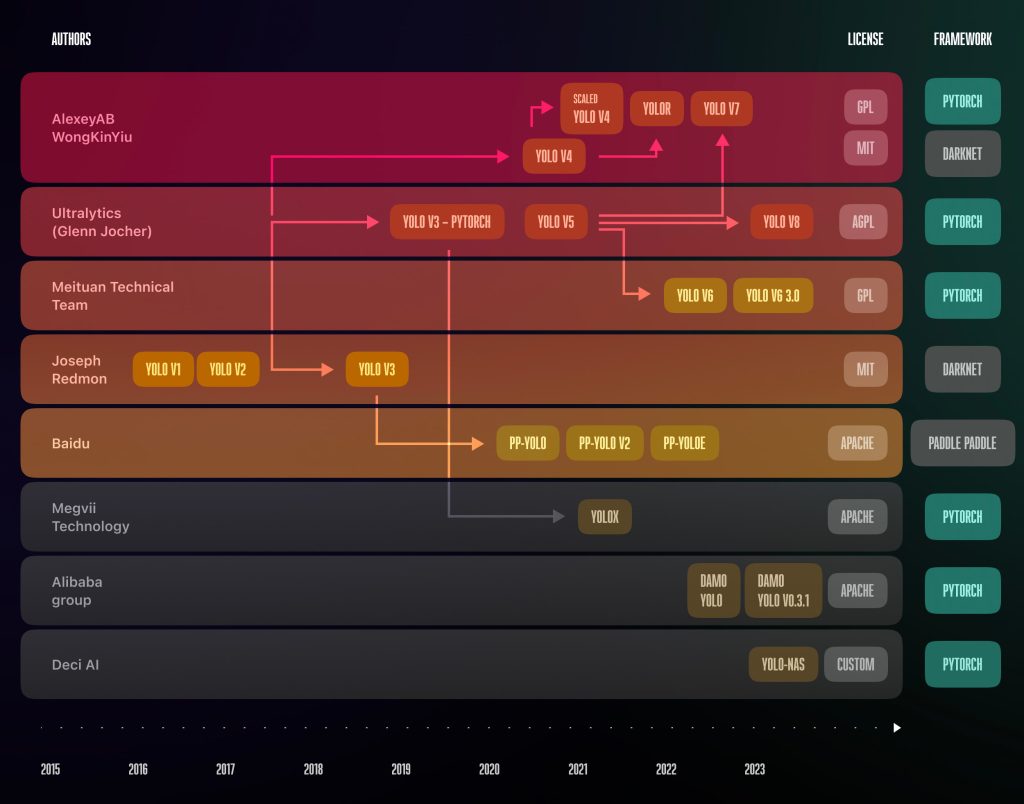
Image Credit : OpenCV.ai
📜 Licensing Overview:
- MIT License: Used in the early YOLO versions, allowing for broad use and modification with few restrictions.
- GPL License: Encourages collaboration while ensuring that derivative work remains open source.
- Apache License: Offers a balance between open-source freedom and commercial use, widely adopted in enterprise environments.
💡 Frameworks:
- Darknet: The original framework used in early YOLO versions, known for its speed and efficiency.
- PyTorch: Dominates recent YOLO models, providing flexibility and a rich ecosystem for development and deployment.
- PaddlePaddle: Baidu’s in-house framework, optimized for PP-YOLO models, ensuring tight integration with Baidu’s AI infrastructure.
As we continue to push the boundaries of AI, the evolution of YOLO to what it is today has been of immense help to computer vision developers. Each new iteration not only refines performance but also expands the possibilities of what AI can achieve in real-world applications. Whether you’re developing on the edge or deploying in the cloud, the YOLO family offers a model for every need. 🌍